Table Of Content
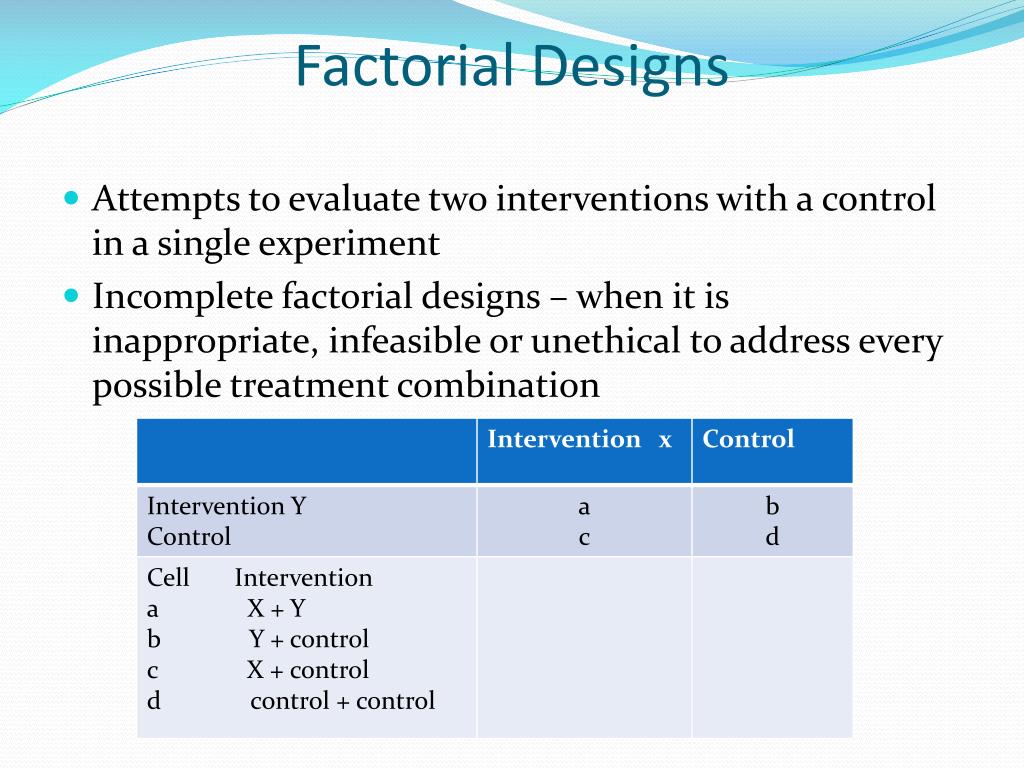
The outcomes for drug A are compared to those on drug A, drug A and B and to those who were on drug B and neither drug A nor drug B.15 The advantages of this study design that it saves time and helps to study two different drugs on the same study population at the same time. However, this study design would not be applicable if either of the drugs or interventions overlaps with each other on modes of action or effects, as the results obtained would not attribute to a particular drug or intervention. From an epidemiological standpoint, there are two major types of clinical study designs, observational and experimental.3 Observational studies are hypothesis‐generating studies, and they can be further divided into descriptive and analytic. Descriptive observational studies provide a description of the exposure and/or the outcome, and analytic observational studies provide a measurement of the association between the exposure and the outcome. It involves an intervention that tests the association between the exposure and outcome. Each study design is different, and so it would be important to choose a design that would most appropriately answer the question in mind and provide the most valuable information.
Lesson 5: Introduction to Factorial Designs
Optimization of harvest and extraction factors by full factorial design for the improved yield of C-glucosyl xanthone ... - Nature.com
Optimization of harvest and extraction factors by full factorial design for the improved yield of C-glucosyl xanthone ....
Posted: Wed, 11 Aug 2021 07:00:00 GMT [source]
Community trials are also known as cluster‐randomized trials, involve groups of individuals with and without disease who are assigned to different intervention/experiment groups. Some of the biases observed with cohort studies include selection bias and information bias. Some individuals who have the exposure may refuse to participate in the study or would be lost to follow‐up, and in those instances, it becomes difficult to interpret the association between an exposure and outcome. Also, if the information is inaccurate when past records are used to evaluate for exposure status, then again, the association between the exposure and outcome becomes difficult to interpret. One of the aspects that is often overlooked is the selection of cases and controls.
Factorial experiment
Using the Taguchi experimental design for assessing within-field variability of surface run-off and soil erosion risk - ScienceDirect.com
Using the Taguchi experimental design for assessing within-field variability of surface run-off and soil erosion risk.
Posted: Fri, 01 Jul 2022 07:00:00 GMT [source]
You have been employed by SuperGym, a local personal training gym, who want an engineer's perspective on how to offer the best plans to their clients. SuperGym currently categorizes her clients into 4 body types to help plan for the best possible program. In the "Modify Design" menu, users can modify factors, replicate design, randomize design, renumber design, fold design, and add axial points. To change the factors, click the "Modify factors" radio button and then "Specify" to see the following options menu.
Book traversal links for Lesson 5: Introduction to Factorial Designs
This can be conducted with or without replication, depending on its intended purpose and available resources. It will provide the effects of the three independent variables on the dependent variable and possible interactions. Suppose you have two variables \(A\) and \(B\) and each have two levels a1, a2 and b1, b2.
For example, a researcher might choose to treat cell phone use as a within-subjects factor by testing the same participants both while using a cell phone and while not using a cell phone. But they might choose to treat time of day as a between-subjects factor by testing each participant either during the day or during the night (perhaps because this only requires them to come in for testing once). Thus each participant in this mixed design would be tested in two of the four conditions. In the remainder of this section, we will focus on between-subjects factorial designs only.
How to Deal with a 2n Factorial Design
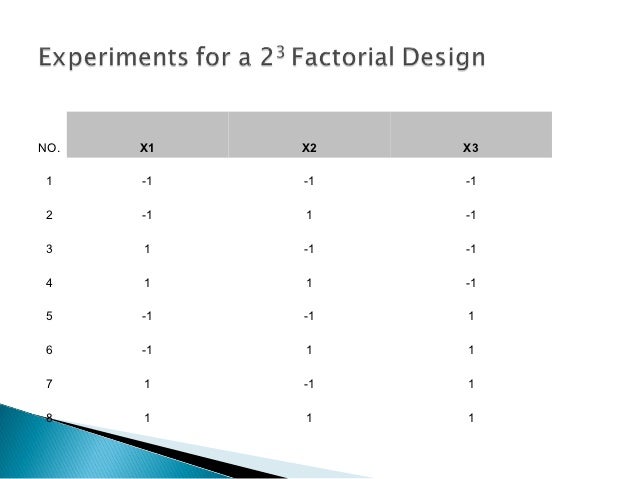
Thus it is important to be aware of which variables in a study are manipulated and which are not. First, non-manipulated independent variables are usually participant characteristics (private body consciousness, hypochondriasis, self-esteem, and so on), and as such they are, by definition, between-subject factors. For example, people are either low in hypochondriasis or high in hypochondriasis; they cannot be in both of these conditions. Recall that in a simple between-subjects design, each participant is tested in only one condition. In a simple within-subjects design, each participant is tested in all conditions. In a factorial experiment, the decision to take the between-subjects or within-subjects approach must be made separately for each independent variable.
When researchers combine dependent variables in this way, they are treating them collectively as a multiple-response measure of a single construct. The advantage of this is that multiple-response measures are generally more reliable than single-response measures. However, it is important to make sure the individual dependent variables are correlated with each other by computing an internal consistency measure such as Cronbach’s \(\alpha\). If they are not correlated with each other, then it does not make sense to combine them into a measure of a single construct. If they have poor internal consistency, then they should be treated as separate dependent variables. A non‐randomized clinical trial involves an approach to selecting controls without randomization.
2.2. Factorial Designs¶
They found that neither religiosity nor ethnicity was correlated with generosity and were therefore able to rule them out as third variables. This does not prove that SES causes greater generosity because there could still be other third variables that the researchers did not measure. But by ruling out some of the most plausible third variables, the researchers made a stronger case for SES as the cause of the greater generosity. The second way of looking at the interaction is to start by looking at the other variable.
In statistics, a full factorial experiment is an experiment whose design consists of two or more factors, each with discrete possible values or "levels", and whose experimental units take on all possible combinations of these levels across all such factors. Such an experiment allows the investigator to study the effect of each factor on the response variable, as well as the effects of interactions between factors on the response variable. Interactions occur when the effect of an independent variable depends on the levels of the other independent variable.
Thus, this experiment would address which type of NRT exerts additive or interactive effects when used with varenicline, and whether the adherence intervention exerts main or interactive effects. Obviously the investigator must make the intervention relevant to each medication type, but such adjustment raises questions. Is the adherence intervention different enough in its various forms (across medications) so that it no longer constitutes a single, coherent component? If that is true, its effects cannot be interpreted in a straightforward manner.
These different formats can make the data look different, even though the pattern in the data is the same. An important skill to develop is the ability to identify the patterns in the data, regardless of the format they are presented in. Some examples of bar and line graphs are presented in the margin, and two example tables are presented below. To find the main effect of the shoes manipulation we want to find the mean height in the no shoes condition, and compare it to the mean height of the shoes condition.
This can be conceptualized as a 2 × 2 factorial design with mood (positive vs. negative) and self-esteem (high vs. low) as non-manipulated between-subjects factors. But factorial designs can also include only non-manipulated independent variables, in which case they are no longer experiments but are instead non-experimental in nature. But factorial designs can also include only non-manipulated independent variables, in which case they are no longer experiment designs, but are instead non-experimental in nature. Such studies are extremely common, and there are several points worth making about them.
The different ICs when used in real world settings would entail different amounts of contact or different delivery routes and their net real world effects would reflect these influences. Thus, it is important to recognize that such effects do not really constitute experimental artifacts, but rather presage the costs of complex treatments as used in real world application, presumably something worth knowing. The choice of control conditions can also affect burden and complexity for both staff and patients. In this regard, “off” conditions (connoting a no-treatment control condition as one level of a factor) have certain advantages. They are relatively easy to implement, they do not add burden to the participants, and they should maximize sensitivity to experimental effects (versus a low-treatment control). Of course, less intensive (versus no-treatment) control conditions might be used for substantive reasons or because they ensure that every participant gets at least some treatment.
However, conducting an RCT that comprises ICs whose joint effects are unknown, poses clear risks. This is because research shows that the effectiveness of a IC can be substantially modulated by the other ICs with which it is used (Cook et al., 2016; Fraser et al., 2014; Schlam et al., 2016); i.e., they may interact. First, non-manipulated independent variables are usually participant background variables (self-esteem, gender, and so on), and as such, they are by definition between-subjects factors. For example, people are either low in self-esteem or high in self-esteem; they cannot be tested in both of these conditions.
No comments:
Post a Comment